Predicting NEET status for the Moroccan young men and women with Random Forest and C50 classifiers.
Conference
64th ISI World Statistics Congress - Ottawa, Canada
Format: CPS Paper
Keywords: boosting, c50, classification, gender, imbalanced, imputation, mice, neet, pruning, random forest, sdgs, smote_nc, undersampling, youth
Abstract
The Moroccan youth population aged from 15 to 29 years old is characterised by significant gender related disparities with respect to NEET (Not in Employment, Education or Training) status. Almost 8 young NEET out of 10 are women, moreover, more than 52% of young women fall in NEET category (most of them are out of labour force housewives), while the percentage of young men who are NEET does not exceed 14% (most of them are unemployed). Within the 2030 Agenda for Sustainable Development framework, the NEET rate has to be substantially reduced; in this context, the present paper tries to draw a clear picture of these vulnerable categories of the population to policy designers in Morocco for better targeting, by separately classifying NEET status of young men and young women using Random Forest and C50 classifiers. To this end, a number of predictors, that were demonstrated to determine in different ways the likelihood of falling in NEET situation based on a previous study using logistic regression, are used in the models; such as age, the level of education, the matrimonial status (it is the variable that best splits the data in C50), the household size, the activity status of the head of household, etc. Besides, preparing the data for the classifiers requires different techniques, namely imputation of missing data using MICE (Multivariate Imputation with Certain Estimations) and balancing the data using under sampling and SMOTE_NC (Synthetic Minority Over-sampling Technique-Nominal Continuous) are applied.
Figures/Tables
RFW1 ERR.rate by ntrees

C50 treeW1

Table1 . List of variables used for building the classifiers
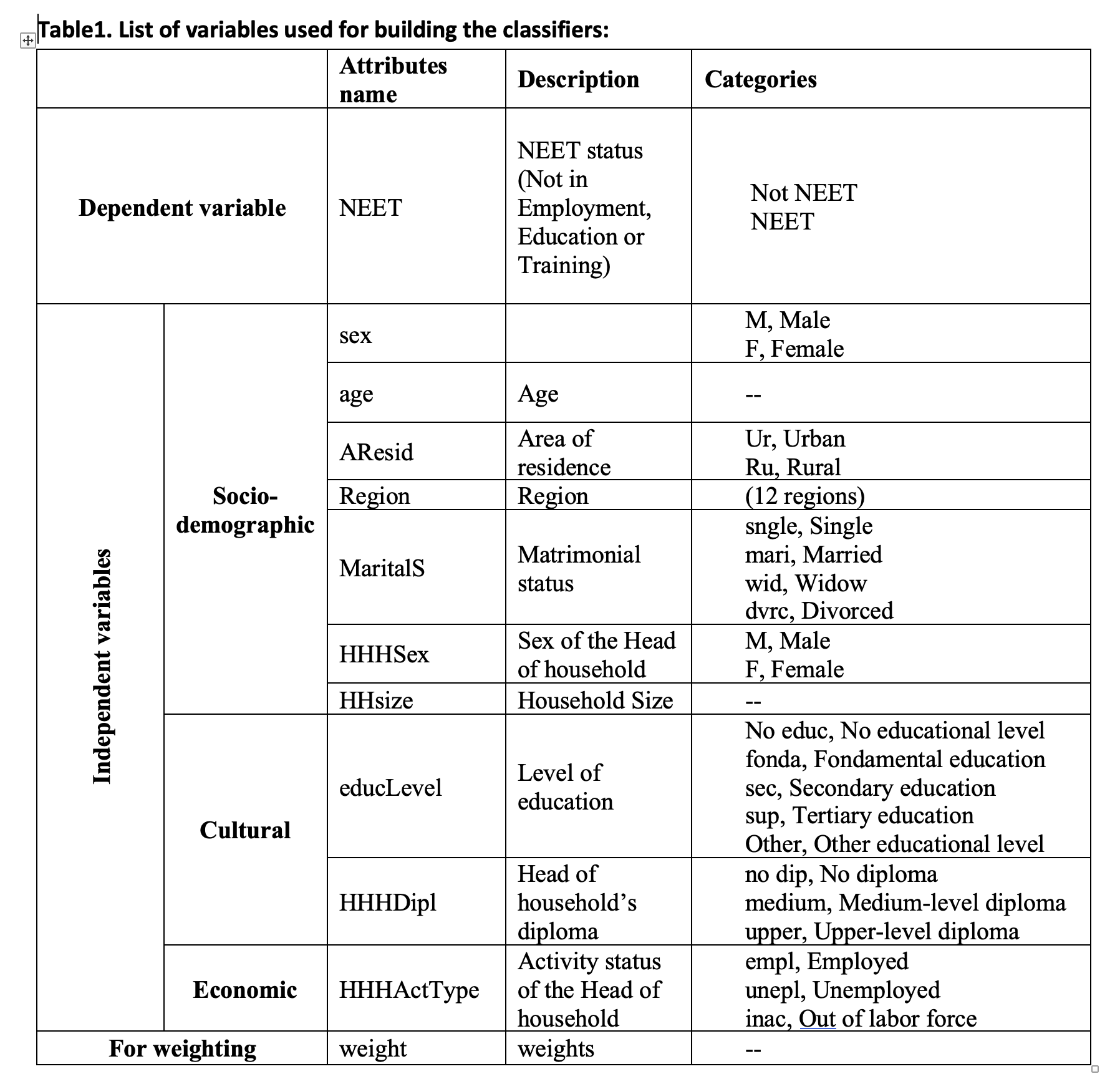
C50 treeM1.4, undersampled data

missing data pattern.dataM
